Neural Strands: Learning Hair Geometry
and Appearance from Multi-View Images
ECCV 2022
Abstract
We present Neural Strands, a novel learning framework for modeling accurate hair geometry and appearance from multi-view image inputs. The learned hair model can be rendered in real-time from any viewpoint with high-fidelity view-dependent effects. Our model achieves intuitive shape and style control unlike volumetric counterparts. To enable these properties, we propose a novel hair representation based on a neural scalp texture that encodes the geometry and appearance of individual strands at each texel location. Furthermore, we introduce a novel neural rendering framework based on rasterization of the learned hair strands. Our neural rendering is strand-accurate and anti-aliased, making the rendering view-consistent and photorealistic. Combining appearance with a multi-view geometric prior, we enable, for the first time, the joint learning of appearance and explicit hair geometry from a multi-view setup. We demonstrate the efficacy of our approach in terms of fidelity and efficiency for various hairstyles.
Video
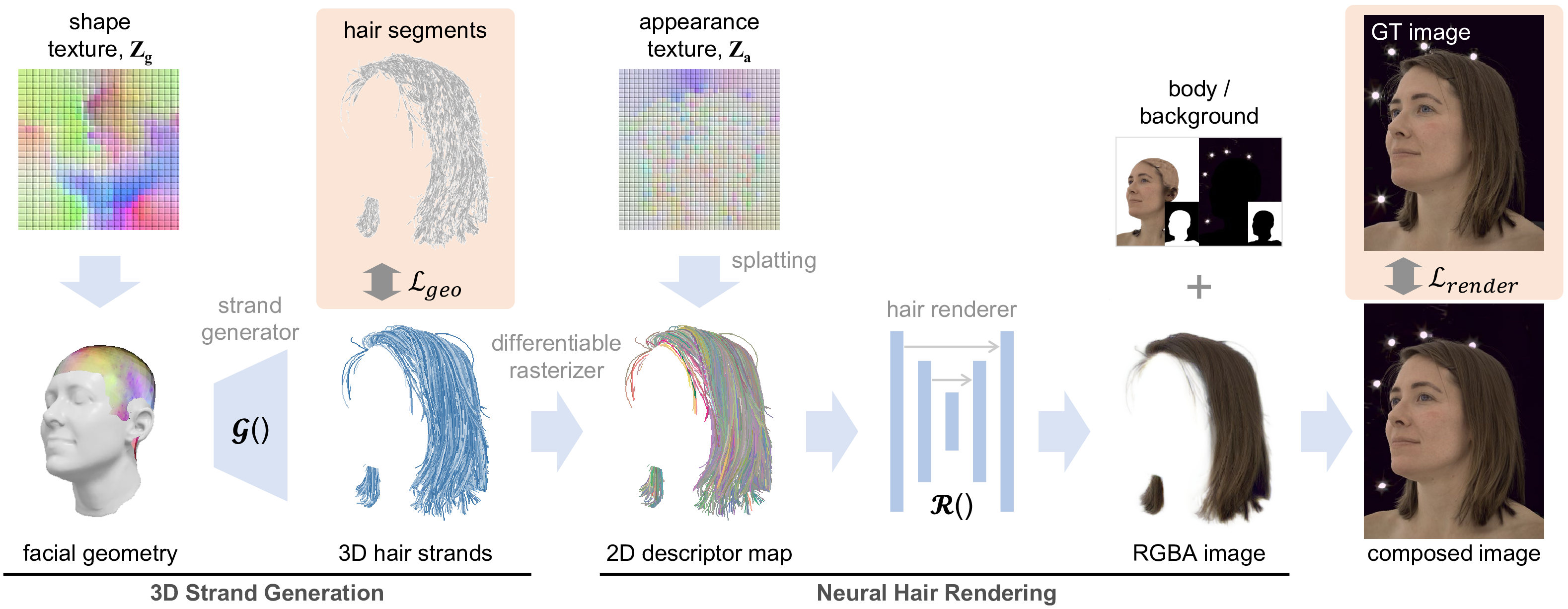
Editability
Our system recovers strand-based geometry together with appearance. This explicit geometry allows for direct editing of the hair and creation of hair-in-wind effects or a virtual haircut. This is in strong contrast to volumetric approaches which do not allow modification of the hair geometry.
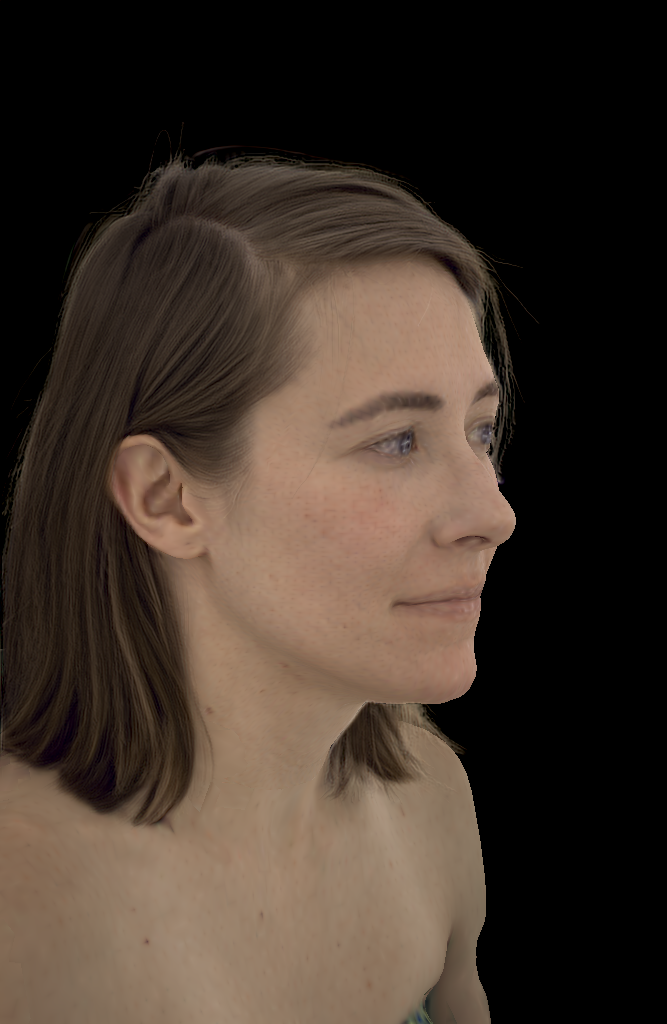
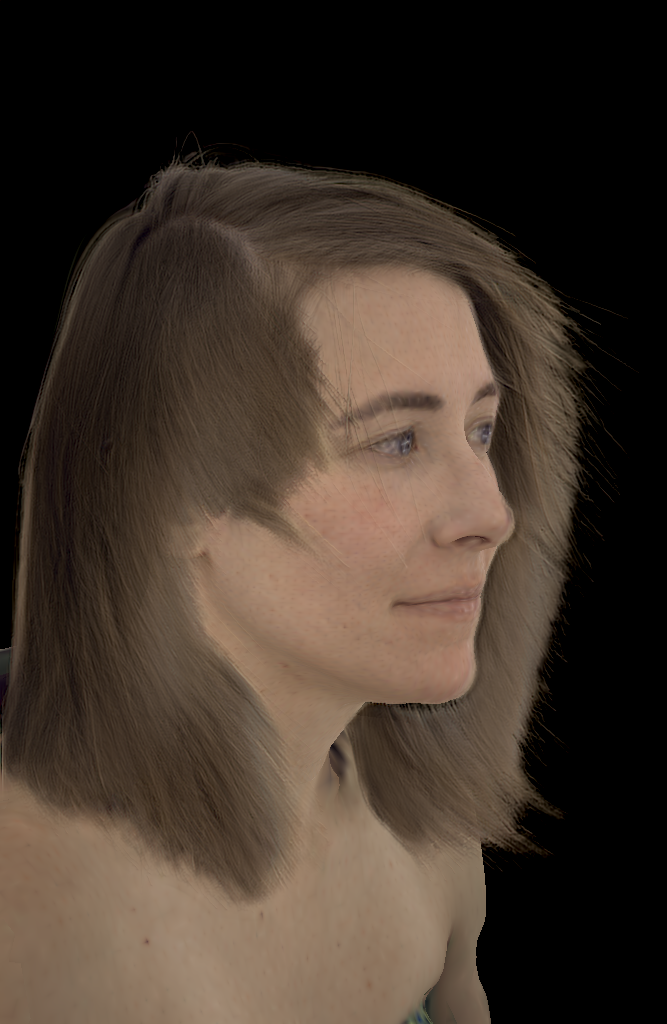
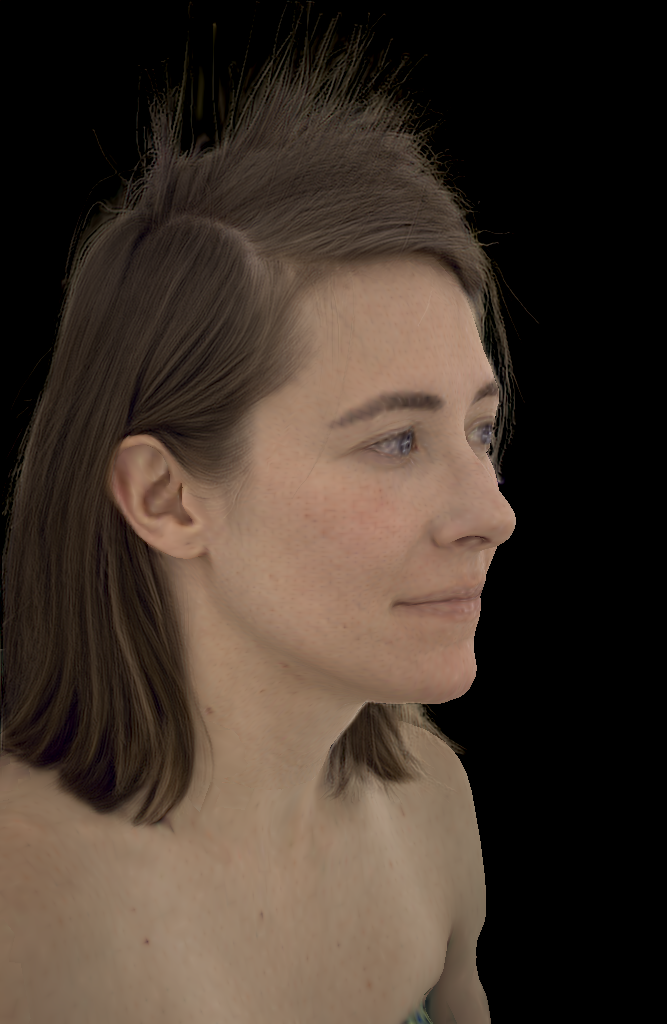
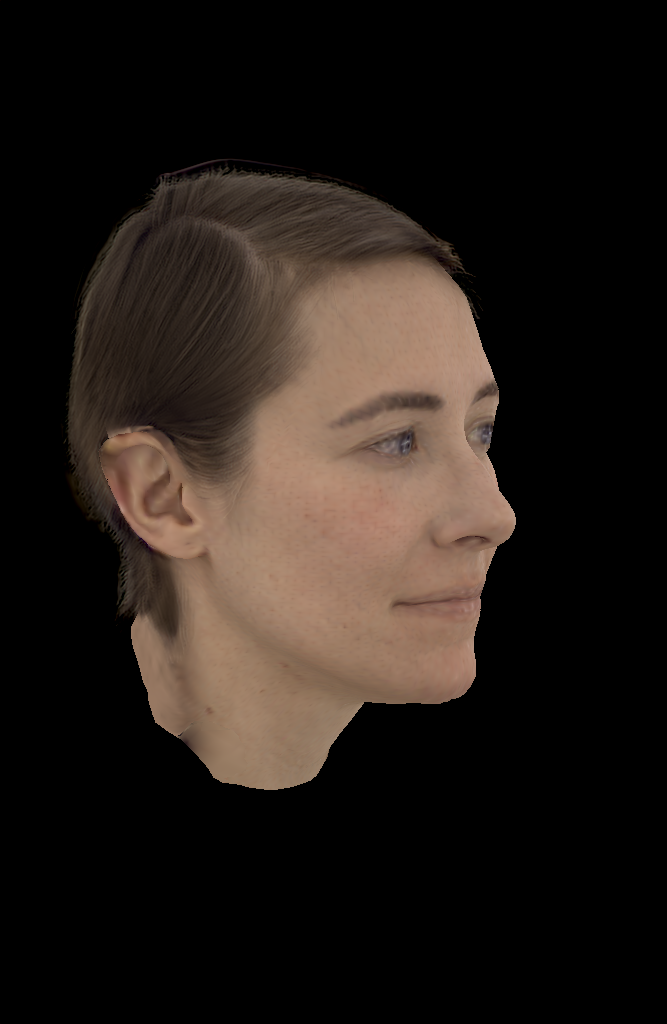
Comparison
NeRF and MVP(Mixture of Volumetric Primitives) fail to recover high frequency detail on the hair. Our method recovers more detailed hair together with strand geometry even for stray-away hairs.
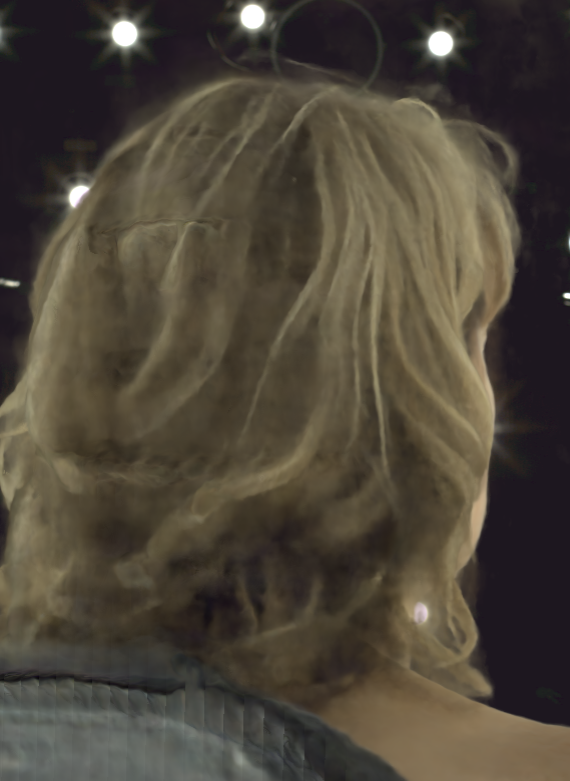
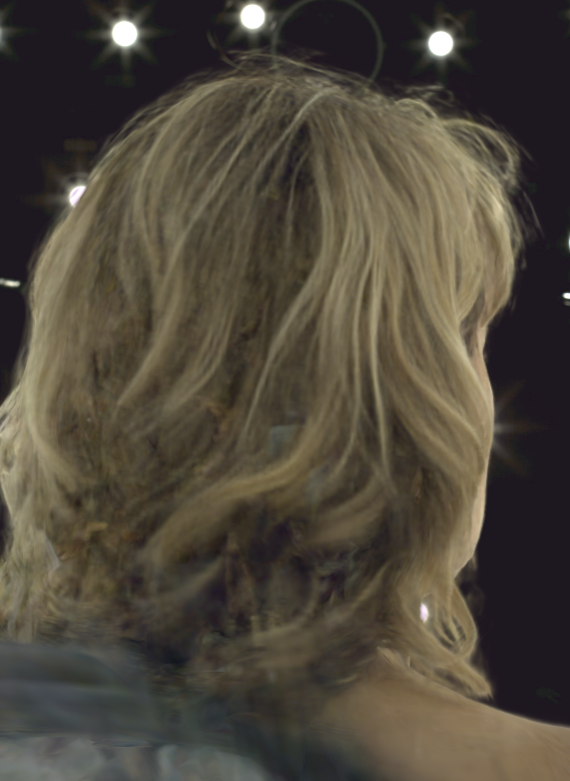
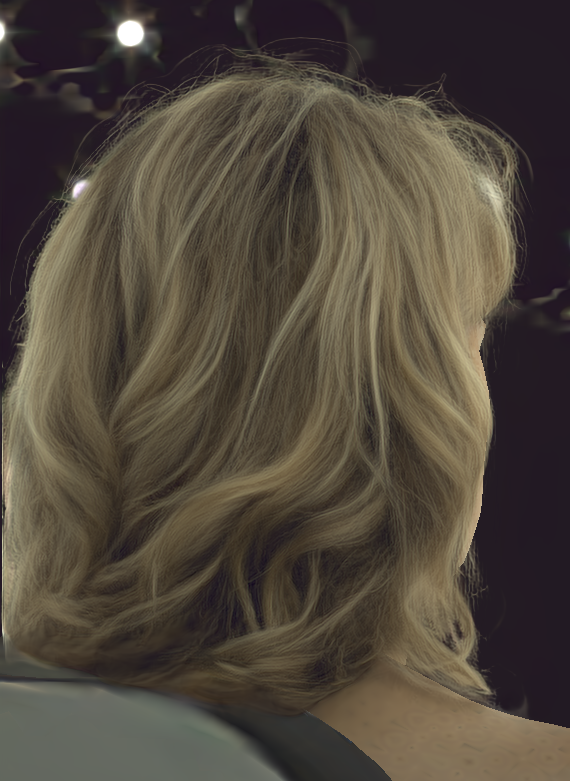
Citation
Acknowledgements
The website template was borrowed from Michaël Gharbi.